使Twitter數(shù)據(jù)對可樂進(jìn)行客戶情感分析
介紹可口可樂(Coca-Cola)和百事可樂(PepsiCo)是軟飲料行業(yè)的知名品牌,兩家公司均躋身《財富》500強(qiáng)。在競爭激烈的市場中擁有廣泛產(chǎn)品線的公司彼此之間存在著激烈的競爭,并在隨后的幾乎所有垂直產(chǎn)品市場中不斷爭奪市場份額。通過從每家公司的官方推特下載5000條推文來分析這兩家公司的客戶情緒,并在R中進(jìn)行分析。在這一分析中,我們可以了解如何從品牌的社交媒體參與(在本例中為推特)中分析客戶情緒。目錄涉及的軟件包及其應(yīng)用什么是情緒分析?清除文本詞云在一天和一周內(nèi)發(fā)布推文推特數(shù)據(jù)的情感評分客戶推特的情感分析結(jié)論R中使用的軟件包
什么是情緒分析?情感分析是一種文本挖掘技術(shù),它為文本提供上下文,能夠從主觀抽象的源材料中理解信息,借助Facebook、Instagram等社交媒體平臺上的在線對話,幫助理解對品牌產(chǎn)品或服務(wù)的社會情感,推特或電子郵件。眾所周知,計算機(jī)不理解我們的通用語言,為了讓他們理解自然語言,我們首先將單詞轉(zhuǎn)換成數(shù)字格式。接下來我們將嘗試一步一步地去實現(xiàn)這一過程。清除文本我們已經(jīng)從Twitter下載了數(shù)據(jù)集,由于推特的文本形式包含了鏈接、hashtags、推特er句柄名稱和表情符號,為了刪除它們,我們在R中編寫了函數(shù)ions。刪除這些無用信息后,所有文本都將轉(zhuǎn)換為小寫,刪除英語中沒有意義的停止詞(如冠詞、介詞等)、標(biāo)點符號和數(shù)字,然后再將它們轉(zhuǎn)換為文檔術(shù)語矩陣。文檔術(shù)語矩陣:是一個矩陣,包含每個單詞在每個文檔上出現(xiàn)的次數(shù)。removeURL <- function(x) gsub(“(f|ht)tp(s?)://S+”, “”, x, perl=T)
removeHashTags <- function(x) gsub(“#S+”, “”, x)
removeTwitterHandles <- function(x) gsub(“@S+”, “”, x)
removeSlash <- function(x) gsub(“n”,” “, x)
removeEmoticons <- function(x) gsub(“[^x01-x7F]”, “”, x)
data_pepsi$text <- iconv(data_pepsi$text, to = “utf-8”)
pepsi_corpus <- Corpus(VectorSource(data_pepsi$text))
pepsi_corpus <- tm_map(pepsi_corpus,tolower)
pepsi_corpus <- tm_map(pepsi_corpus,removeWords,stopwords(“en”))
pepsi_corpus <- tm_map(pepsi_corpus,content_transformer(removeHashTags))
pepsi_corpus <- tm_map(pepsi_corpus,content_transformer(removeTwitterHandles))
pepsi_corpus <- tm_map(pepsi_corpus,content_transformer(removeURL))
pepsi_corpus <- tm_map(pepsi_corpus,content_transformer(removeSlash))
pepsi_corpus <- tm_map(pepsi_corpus,removePunctuation)
pepsi_corpus <- tm_map(pepsi_corpus,removeNumbers)
pepsi_corpus <- tm_map(pepsi_corpus,content_transformer(removeEmoticons))
pepsi_corpus <- tm_map(pepsi_corpus,stripWhitespace)
pepsi_clean_df <- data.frame(text = get(“content”, pepsi_corpus))
dtm_pepsi <- DocumentTermMatrix(pepsi_corpus)
dtm_pepsi <- removeSparseTerms(dtm_pepsi,0.999)
pepsi_df <- as.data.frame(as.matrix(dtm_pepsi))
data_cola$text <- iconv(data_cola$text, to = “utf-8”)
cola_corpus <- Corpus(VectorSource(data_cola$text))
cola_corpus <- tm_map(cola_corpus,tolower)
cola_corpus <- tm_map(cola_corpus,removeWords,stopwords(“en”))
cola_corpus <- tm_map(cola_corpus,content_transformer(removeHashTags))
cola_corpus <- tm_map(cola_corpus,content_transformer(removeTwitterHandles))
cola_corpus <- tm_map(cola_corpus,content_transformer(removeURL))
cola_corpus <- tm_map(cola_corpus,content_transformer(removeSlash))
cola_corpus <- tm_map(cola_corpus,removePunctuation)
cola_corpus <- tm_map(cola_corpus,removeNumbers)
cola_corpus <- tm_map(cola_corpus,content_transformer(removeEmoticons))
cola_corpus <- tm_map(cola_corpus,stripWhitespace)
cola_clean_df <- data.frame(text = get(“content”, cola_corpus))
dtm_cola <- DocumentTermMatrix(cola_corpus)
dtm_cola <- removeSparseTerms(dtm_cola,0.999)
cola_df <- as.data.frame(as.matrix(dtm_cola))
詞云wordcloud是測試數(shù)據(jù)的一種表示形式,它通過增加測試數(shù)據(jù)的大小來突出顯示最常用的單詞,該技術(shù)用于將文本可視化為圖像,是單詞或標(biāo)簽的集合。在R中,可以使用worldcloud2包來實現(xiàn),以下是它的輸出代碼。word_pepsi_df <- data.frame(names(pepsi_df),colSums(pepsi_df))
names(word_pepsi_df) <- c(“words”,”freq”)
word_pepsi_df <- subset(word_pepsi_df, word_pepsi_df$freq > 0)
wordcloud2(data = word_pepsi_df,size = 1.5,color = “random-light”,backgroundColor = “dark”)
word_cola_df <- data.frame(names(cola_df),colSums(cola_df))
names(word_cola_df) <- c(“words”,”freq”)
word_cola_df <- subset(word_cola_df, word_cola_df$freq > 0)
wordcloud2(data = word_cola_df,size = 1.5,color = “random-light”,backgroundColor = “dark”)
百事可樂和可口可樂的推特數(shù)據(jù)的詞云
正如我們所知,詞云中的詞大小取決于其在推特中的頻率,因此詞會不斷變化, just, native, right, racism很多出現(xiàn)在百事可樂客戶的推特中,而get和support等詞更多地出現(xiàn)在可口可樂客戶的推特中。在一天和一周內(nèi)發(fā)布推文由于推特收集的時間跨度超過一周,因此我們可以分析大多數(shù)用戶活躍或用戶在該品牌上發(fā)布最多推文的時間和工作日,這可以通過使用ggplot2庫的折線圖來可視化。下面是與輸出一起使用的函數(shù)data_pepsi$Date <- as.Date(data_pepsi$created_at)
data_pepsi$hour <- hour(data_pepsi$created_at)
data_pepsi$weekday<-factor(weekdays(data_pepsi$Date),levels=c(“Monday”,”Tuesday”,”Wednesday”,”Thursday”,”Friday”,”Saturday”,”Sunday”))
ggplot(data_pepsi,aes(x= hour)) + geom_density() + theme_minimal() + ggtitle(“Pepsi”)
ggplot(data_pepsi,aes(x= weekday)) + geom_bar(color = “#CC79A7”, fill = “#CC79A7”) + theme_minimal() +ggtitle(“Pepsi”) + ylim(0,1800)
data_cola$Date <- as.Date(data_cola$created_at)
data_cola$Day <- day(data_cola$created_at)
data_cola$hour <- hour(data_cola$created_at)
data_cola$weekday<-factor(weekdays(as.Date(data_cola$Date)),levels=c(“Monday”,”Tuesday”,”Wednesday”,”Thursday”,”Friday”,”Saturday”,”Sunday”))
ggplot(data_cola,aes(x= hour)) + geom_density() + theme_minimal() + ggtitle(“Coca-Cola”)
ggplot(data_cola,aes(x=
weekday)) + geom_bar(color = “#CC79A7”, fill = “#CC79A7”) + theme_minimal()
從上面的圖表中,我們可以看到百事可樂和可口可樂在下午3-4點和凌晨1點左右都出現(xiàn)了峰值,因為人們喜歡在工作無聊或深夜使用社交媒體,這在我們的工作中是顯而易見的。
一周內(nèi)推特的分布情況
當(dāng)每日推文顯示在條形圖上時,對于百事來說,周四是推特數(shù)量最多的一天,這是因為他們發(fā)布了季度報告,但就可口可樂而言,周二我們看到的推特數(shù)量最少。推特數(shù)據(jù)的情感評分在本節(jié)中,我們把推特數(shù)據(jù)分為積極的、消極的和中立的,這可以通過使用sendimentR包來實現(xiàn),該軟件包為每個詞典單詞分配一個從-1到+1的情感評分,并取推特中每個單詞的平均值,得到每個推特的最終情感評分。sentiments <- sentiment_by(get_sentences(pepsi_clean_df$text))
data$sentiment_score <- round(sentiments$ave_sentiment,2)
data$sentiment_score[data_pepsi$sentiment_score > 0] <- “Positive”
data$sentiment_score[data_pepsi$sentiment_score < 0] <- “Negative”
data$sentiment_score[data_pepsi$sentiment_score == 0] <- “Neutral”
data$sentiment_score <- as.factor(data$sentiment_score)
ggplot(data,aes(x = sentiment_score)) + geom_bar(color = “steelblue”, fill = “steelblue”) + theme_minimal()
幾乎75%的推特用戶都持肯定態(tài)度,因為這兩個品牌在他們的客戶中相當(dāng)受歡迎。顧客推特的情感分析推特的情緒是由Syuzhet軟件包執(zhí)行的,該軟件包根據(jù)十個情緒指數(shù)對每個詞典單詞進(jìn)行評分,包括憤怒、預(yù)期、厭惡、恐懼、喜悅、悲傷、驚訝、信任、消極和積極。如果我們把索引上每個詞的值加起來,所有推特的情緒都可以用條形圖表示。cols <- c(“red”,”pink”,”green”,”orange”,”yellow”,”skyblue”,”purple”,”blue”,”black”,”grey”)
pepsi_sentimentsdf <- get_nrc_sentiment(names(pepsi_df))
barplot(colSums(pepsi_sentimentsdf),
main = “Pepsi”,col = cols,space = 0.05,horiz = F,angle = 45,cex.a(chǎn)xis = 0.75,las = 2,srt = 60,border = NA)
cola_sentimentsdf <- get_nrc_sentiment(names(cola_df))
barplot(colSums(cola_sentimentsdf),
main = “Coca-Cola”,col = cols,space = 0.05,horiz = F,angle = 45,cex.a(chǎn)xis = 0.75,las = 2,srt = 60,border = NA)
上面的輸出是所有情緒在條形圖上的顯示,因為從條形圖可以很清楚地看出,積極性對兩家公司都起主導(dǎo)作用,這進(jìn)一步加強(qiáng)了我們的上述假設(shè)。繼續(xù)跟蹤圖表中的變化可以作為對新產(chǎn)品或廣告的反饋。最常用詞word_pepsi_df$words <- factor(word_pepsi_df$words, levels = word_pepsi_df$words[order(word_pepsi_df$freq)])
word_cola_df$words <- factor(word_cola_df$words, levels = word_cola_df$words[order(word_cola_df$freq)])
ggplot(word_pepsi_df[1:15,],aes(x = freq, y = words)) + geom_bar(stat = “identity”, color = “#C4961A”,fill = “#C4961A”) + theme_minimal() + ggtitle(“Pepsi”)
ggplot(word_cola_df[1:15,],aes(x = freq, y = words)) + geom_bar(stat = “identity”, color = “#C4961A”,fill = “#C4961A”) + theme_minimal() + ggtitle(“Coca-Cola”)
createNgram <-function(stringVector, ngramSize){
ngram <- data.table()
ng <- textcnt(stringVector, method = “string”, n=ngramSize, tolower = FALSE)
if(ngramSize==1){
ngram <- data.table(w1 = names(ng), freq = unclass(ng), length=nchar(names(ng)))
}
else {
ngram <- data.table(w1w2 = names(ng), freq = unclass(ng), length=nchar(names(ng)))
}
return(ngram)
}
pepsi_bigrams_df <- createNgram(pepsi_clean_df$text,2)
cola_bigrams_df <- createNgram(cola_clean_df$text,2)
pepsi_bigrams_df$w1w2 <- factor(pepsi_bigrams_df$w1w2,levels = pepsi_bigrams_df$w1w2[order(pepsi_bigrams_df$freq)])
cola_bigrams_df$w1w2 <- factor(cola_bigrams_df$w1w2,levels = cola_bigrams_df$w1w2[order(cola_bigrams_df$freq)])
names(pepsi_bigrams_df) <- c(“words”, “freq”, “l(fā)ength”)
names(cola_bigrams_df) <- c(“words”, “freq”, “l(fā)ength”)
ggplot(pepsi_bigrams_df[1:15,],aes(x = freq, y = words)) + geom_bar(stat = “identity”, color = “#00AFBB”,fill = “#00AFBB”) + theme_minimal() + ggtitle(“Pepsi”)
ggplot(cola_bigrams_df[1:15,],aes(x = freq, y = words)) + geom_bar(stat = “identity”, color = “#00AFBB”,fill = “#00AFBB”) + theme_minimal() + ggtitle(“Coca-Cola”)
二元語法二元語法是一對字詞,當(dāng)句子被拆分成兩個字詞時產(chǎn)生的。獲取單詞的上下文是有用的,因為單個單詞通常不提供任何上下文。
結(jié)論我們可以看到,從現(xiàn)有的社交媒體參與度來看,公司可以分析客戶的情緒,并據(jù)此制定業(yè)務(wù)戰(zhàn)略,來用于制定公司決策(例如啟動產(chǎn)品線)。
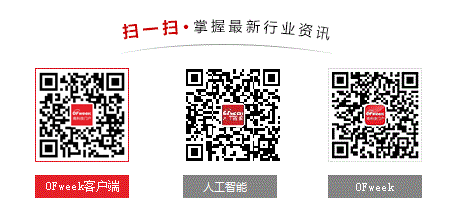
請輸入評論內(nèi)容...
請輸入評論/評論長度6~500個字
最新活動更多
-
10月31日立即下載>> 【限時免費下載】TE暖通空調(diào)系統(tǒng)高效可靠的組件解決方案
-
即日-11.13立即報名>>> 【在線會議】多物理場仿真助跑新能源汽車
-
11月28日立即報名>>> 2024工程師系列—工業(yè)電子技術(shù)在線會議
-
12月19日立即報名>> 【線下會議】OFweek 2024(第九屆)物聯(lián)網(wǎng)產(chǎn)業(yè)大會
-
即日-12.26火熱報名中>> OFweek2024中國智造CIO在線峰會
-
即日-2025.8.1立即下載>> 《2024智能制造產(chǎn)業(yè)高端化、智能化、綠色化發(fā)展藍(lán)皮書》
推薦專題
- 高級軟件工程師 廣東省/深圳市
- 自動化高級工程師 廣東省/深圳市
- 光器件研發(fā)工程師 福建省/福州市
- 銷售總監(jiān)(光器件) 北京市/海淀區(qū)
- 激光器高級銷售經(jīng)理 上海市/虹口區(qū)
- 光器件物理工程師 北京市/海淀區(qū)
- 激光研發(fā)工程師 北京市/昌平區(qū)
- 技術(shù)專家 廣東省/江門市
- 封裝工程師 北京市/海淀區(qū)
- 結(jié)構(gòu)工程師 廣東省/深圳市